Prediction of pre-service teachers' academic self-efficacy through machine learning approaches
Hatice YildizAfrican Educational Research Journal
Published: January 23 2023
Volume 11, Issue 1
Pages 32-44
DOI: https://doi.org/10.30918/AERJ.111.22.083
Abstract
The aim of this study was to investigate the extent to which pre-service teachers' belief in academic engagement, student burnout, and proactive strategies predicts academic self-efficacy through machine learning approach. The study group consisted of 446 pre-service teachers at Sivas Cumhuriyet University, Faculty of Education. The Academic Self-Efficacy Scale, Academic Involvement Scale, Maslach Burnout Inventory-Student Scale, and Proactive Strategy Scale were used for data collection. In data analysis, two different machine learning approaches were used; linear regression and artificial neural networks (ANNs). As a result of the regression analysis, a positive, and significant relationship was found between the academic self-efficacy of pre-service teachers, their academic engagement, and proactive strategy. Also, there was a negative and significant relationship between pre-service teachers' academic self-efficacy and academic burnout. Considering the results of the regression analysis, academic engagement, academic burnout, and proactive strategy together explained 38% of academic self-efficacy. When the ANNs results were examined, it was seen that these three variables explained 77% of academic self-efficacy. Therefore, it was understood that ANNs perform better than multiple regression in predicting academic self-efficacy.
Keywords: Academic self-efficacy, academic engagement, student burnout, proactive strategies, multiple regression, artificial neural networks.
Full Text PDFThis article is published under the terms of the Creative Commons Attribution License 4.0
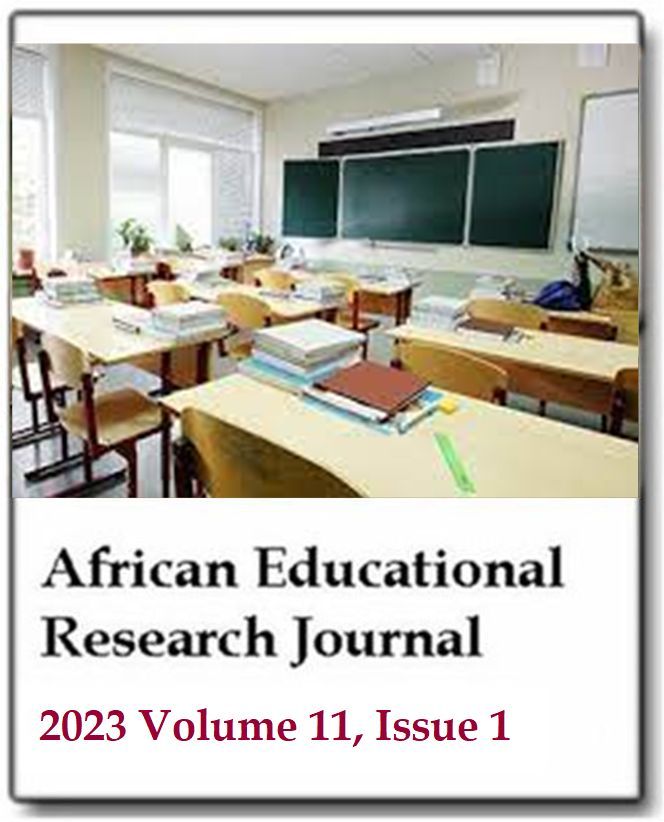